Eric Moulines: EURASIP 2020 Technical Achievement Award
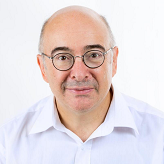
The European Association for Signal Processing (EURASIP) is a prestigious learned society that awards a prize each year to a researcher who has made a mark on the discipline through his work, like Martin Vetterli, current President of the Swiss Federal Institute of Technology in Lausanne (EPFL).
This year, this distinction is awarded to Éric Moulines, professor at the Center for Applied Mathematics (CMAP*) for his work in statistical inference, in particular on hidden Markov chains and non-linear filtering.
"I was very pleasantly surprised by this prize, which shows that the signal processing community still considers me as one of them," explains the researcher. Indeed, Éric Moulines' astonishment was prompted by a change in career direction that occurred some fifteen years ago towards computational statistics.
Cutting-edge research in signal processing
"I devoted my early career to signal processing," says Éric Moulines, "and hidden Markov chains, as well as non-linear filtering, were very useful, especially for tracking temporal evolution and trajectography.”
Indeed, mainly used for sound as the researcher began his research on these themes, he extended these concepts to other models such as hydrology (fluctuating water levels in rivers, very useful for climate models), robot localization or tracking objects in complex environments. "At the time, pattern recognition on static images was not as advanced as they are today, so to track an object, it was easier to compare the temporal evolution of the images to detect what was moving.”
"I dedicated my early career to signal processing," says Éric Moulines. "I discovered hidden Markov chains in the mid-1980s. These tools were at the origin of the first "revolution" in speech recognition and opened the way to learning methods. "
The fundamental idea of hidden Markov chains is to postulate the existence of a hidden process - a latent representation -, often relatively simple. This latent process is supposed to give rise to (potentially very complex) observations, seen as random functions of this state. "In the early 1980s, the hidden process was most often a chain with a finite number of states. " explains the researcher. "I actively participated in the generalization of hidden Markov chains to take into account much more complex latent dynamics. With my students, who have now often become colleagues, we have both developed the theoretical basis for the inference of such models, but also proposed very innovative numerical methods: sequential Monte Carlo methods." These approaches allow the development of "non-linear filtering" methods used today in many domains: tracking of objects in complex environments (allowing for example a robot to move in a cluttered environment), navigation systems on digital maps, prediction of time series (hydrology, climate, etc...).
From signal to data
Éric Moulines' career change came about when he changed the subject of his study. From signals, that are mainly physical such as sound, position, pressure, temperature, he became interested in computational statistics and in the processing of what is now called Big Data. In fact, he was already working on large data sets in the field of signal processing, and the transition from one to the other happened naturally.
"The problems we had in signal processing 20 years ago are the ones at the heart of today's statistical learning," he says. "The data are more varied, but the themes, such as modeling temporal structures, data dependency, or uncertainties and latent data are very similar. ».
Éric Moulines is therefore committed to continuing his research, which has now been rewarded by the signal processing community, and to implementing it within HI! Paris, the new Interdisciplinary Center of the Institut Polytechnique de Paris and HEC dedicated to data analysis and artificial intelligence for science, business and society, of which he is the scientific co-director.
Learn more about Éric Moulines
An alumnus of the École Polytechnique (X 1981) and an engineer from the Telecom Corps, Éric Moulines began his career at Télécom ParisTech. From 1996 to 2015, he was a professor there, where he founded the teaching in statistics and data sciences. He joined the École polytechnique in September 2015 to develop these courses at the engineering cycle and master's level, in particular the Master of Science Big Data for Business associating X and HEC.
In 2017, he was appointed to the Academy of Sciences in the Mechanical and Computer Sciences section, of which he is currently the delegate. This appointment confirmed the influence of his work in a field of applied mathematics emerging thanks to Big Data: computational probabilities at the intersection between computational statistics and probabilistic models.
Éric Moulines was also awarded for his work in statistics at the national level by receiving the CNRS Silver Medal in 2010 and the Grand Prix "Orange" of the French Academy of Sciences in 2011. In July 2016, he received international recognition from his peers when he was appointed to the IMS. He is the author of 2 research monographs on inference problems of complex dynamics and more than 100 research papers in international journals. In addition, for three consecutive years between 2013 and 2015, he was the editor-in-chief of Bernoulli, the leading European journal in the field of statistics.
*CMAP: a joint research unit CNRS, École Polytechnique - Institut Polytechnique de Paris