AI Summit: The road to trustworthy Artificial Intelligence
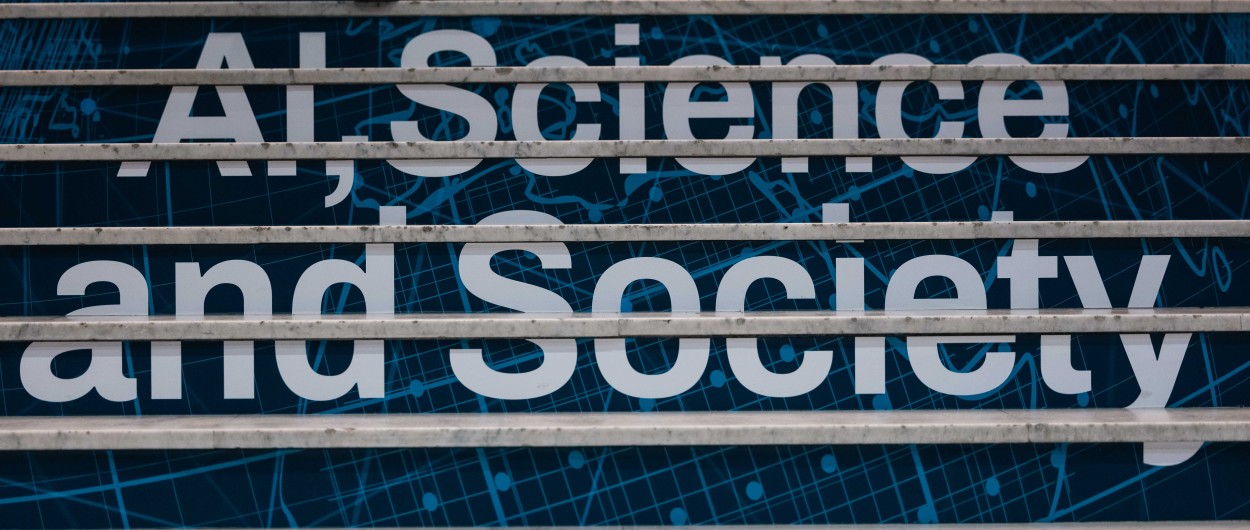
Speakers at the symposium included Aymeric Dieuleveut, Professor at École polytechnique and researcher at the Center for Applied Mathematics (CMAP*), Dame Wendy Hall, Professor of Computer Science at the University of Southampton, Jean-Michel Loubes, Research Director at Inria, Moritz Hardt, Director at the Max Planck Institute for Intelligent Systems and Michael Krajecki, Research Director at the Ministerial Agency for Artificial Intelligence in Defense (AMIAD). The session was moderated by Florence D'Alché-Buc, Professor at Télécom Paris (Institut Polytechnique de Paris).
AI uses are multiplying, sometimes for the better, but with significant risks. Among the harmful social consequences, it is now well documented that AIs can be biased, i.e. their results are systematically (and not accidentally) influenced. Jean-Michel Loubes gave the example of an algorithm making job recommendations from a dataset containing biographical information. By simply changing masculine pronouns to feminine ones, the recommendations change from “surgeons” to “nurses”. These biases can constitute violations of fundamental rights (gender discrimination, racial discrimination, etc.) or have consequences for industry or the economy. For example, an algorithm on a shopping site may systematically favor certain retailers. The biases may come from the dataset used to “train” the AI, or from the algorithm itself.
Tackling biases
In addition to biases, automatic decisions have other consequences, such as self-fulfilling prophecies. Moritz Hardt illustrated this with the Youtube platform, which uses AI to determine which videos to recommend to a user, by estimating how long he or she will stay in front of them. There's a self-fulfilling prophecy effect, as users will actually tend to click on the first recommended video, and therefore spend time on it, making the prediction all the more “true”. The opposite effect, of self-negating prophecy, also exists, for example when the prediction of routes to avoid traffic jams leads to too great an influx of traffic on the proposed route. In other words, predicting something changes the value of what we're trying to predict. AIs therefore have an influential effect, the more powerful the platform. In this case, as in the case of biases, mathematical tools need to be developed to measure these impacts and prevent them. But the challenge of mitigating these effects is not limited to research.
The European Union has adopted recommendations and regulations on AI, in particular the AI act in 2024, as Michael Krajecki pointed out. This text aims to regulate systems commercialized on the European market. In particular, it defines a list of at-risk AI applications, including, for example, systems for recommending job candidates. The AI act establishes responsibilities: model designers must prove compliance, but companies that purchase and deploy an algorithm designed by a third party are also accountable.
A technical and social challenge
One of the requirements for trustworthy AI is respect for the privacy. However, we may find it useful to train certain algorithms on some of our data. Rather than handing over this data to a third party to train an AI system, a new paradigm has emerged over the last ten years: federated learning, as detailed by Aymeric Dieuleveut in his presentation. In this case, the data remains stored locally at the user's end. This type of learning is already implemented in a number of applications, such as when our smartphones suggest the next word to type in an SMS. Aymeric Dieuleveut presented his team's work at École Polytechnique on decentralized architectures, and how they could resist an actor disrupting the learning process.
Beyond the technical aspects, the need for AI to be trustworthy is a societal issue, stressed Dame Wendy Hall. Who establishes how AI should be trusted, and who it should be trusted by? The answer depends on social and political contexts. On a global scale, it is crucial to have a dialogue on this subject, as the UN is trying to do with the United Nations AI Advisory Body. Speakers stressed the importance of supporting academic research, and its independence, as well as the necessary interdisciplinary dimension between mathematics, computer science and social sciences.
*CMAP: a joint research unit CNRS, Inria, École Polytechnique, Institut Polytechnique de Paris, 91120 Palaiseau, France